AI in Banking Risk Management: Popular Use Cases
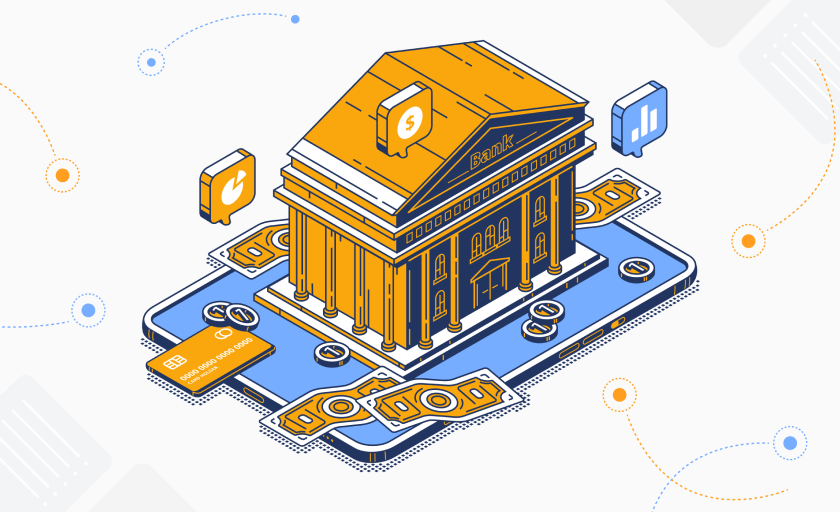
By 2023, the estimated cost savings for banks from AI applications could reach $447 billion. Therefore, it is not surprising that financial institutions are discovering new ways to use artificial intelligence (AI) in their services. From risk management to fraud detection and anti-money laundering, this technology is reshaping how banks operate and paving the way for a more efficient and secure future.
Integrio is passionate about harnessing the power of cutting-edge AI and ML technologies. In this article, we delve into the transformative potential of AI in banking risk management, exploring its diverse applications and the problems it effectively addresses. Join us to uncover how AI revolutionizes banking and advances innovation and efficiency across multiple domains.
Artificial AI in Banking Risk Management
AI-powered risk management for banking combines various aspects of artificial intelligence, machine learning, natural language processing, and other technologies to effectively identify, assess, and mitigate risks.
Artificial Intelligence (AI)
AI involves the development of intelligent systems capable of performing tasks that typically require human intelligence. Risk managers use AI technologies to automate and streamline hazard assessment, fraud detection, and compliance monitoring.
Machine Learning (ML)
ML is all about training algorithms to learn from data and make predictions or decisions. These models are designed to detect fraudulent transactions, evaluate someone's creditworthiness, predict market trends, and identify potential compliance violations.
Deep Learning
Deep learning utilizes artificial neural networks with multiple layers to extract high-level features from raw data. Analyze complex financial data and extract meaningful insights to detect anomalies, predict market fluctuations, or identify potential risks.
Natural Language Processing (NLP)
NLP techniques help analyze unorganized data from customer feedback, regulatory documents, and news articles. It helps make sense of the text and find valuable insights — emerging risks, customer complaints, or regulatory changes.
Big Data Analytics
Big data analytics allows banks to combine internal (transaction records, customer profiles) with external data (market data, social media feeds) to gain a comprehensive view of risks.
Robotic Process Automation (RPA)
RPA allows banks to automate repetitive and rule-based tasks by using software robots, or "bots." In risk management, it helps streamline data entry, data validation, and report generation. By automating these processes, you reduce the chances of manual errors and improve overall operational efficiency.
Crucial Risks in the Banking Industry
The banking sector is using AI and ML to face various risks that can have significant implications for financial institutions. Here are some examples:
Credit Risk
Evaluate potential losses from borrowers or counterparties failing to fulfill their contractual obligations. Credit risk includes the danger of default on loans, bonds, or other credit instruments. It can result from borrowers' financial instability, economic downturns, or inadequate risk assessment and monitoring.
For example, in 2022, the number of insolvent companies in the UK was already 76% higher than in the same period in 2021. This is one of the factors fostering the development of AI in credit risk management.
Market Risk
Market risk arises from adverse charge movements, such as interest or foreign exchange rates, as well as equity and commodity prices. Banks are exposed to it due to their trading activities, investments, and holdings of various financial instruments. Such risks lead to losses if positions are not managed effectively.
To deal with market risks, a global investment bank focuses on redesigning the economic-capital model, the Value-at-Risk (VAR) model, and stress-testing frameworks.
Liquidity Risk
Liquidity risk refers to the inability of a bank to meet its financial obligations as they become due without incurring excessive costs or losses. It occurs when a financial firm experiences difficulty raising funds in the market or faces a sudden surge in demand for withdrawals from depositors. Inadequate liquidity management can threaten a bank's solvency and reputation.
Today, banks have already reduced their liquidity coverage ratios (LCRs) and net stable funding ratios (NSFRs) by 5-20%.
Operational Risk
Operational risk encompasses the danger of losses resulting from inadequate or failed internal processes, systems, human errors, or external events. It includes hazards associated with fraud, cyber attacks, technology failures, legal and regulatory compliance, and disruptions in business operations. As a result, operational risk can lead to financial losses, reputational damage, and legal implications.
In 2021, Envy Group was defrauded of $1.23 billion by a former director, involving fake nickel deals and forged contracts. The current operating status of the company is Liquidation—Compulsory Winding Up (Insolvency).
Compliance and Regulatory Risk
Banks operate in a highly regulated environment, and non-compliance with laws and industry standards can have significant financial and reputational consequences. Compliance and regulatory risks arise from failure to adhere to anti-money laundering (AML) and Know Your Customer (KYC) requirements, data protection regulations, consumer protection laws, and other applicable regulations.
What major issues will be at the top of regulators' minds in 2023? Regulating the use of AI in banking, specialists focus on fulfilling their requirements under the AML Act of 2020 (AMLA), sanctions, and the rising use of digital assets across the banking ecosystem.
Reputational Risk
Reputational risk is the potential loss of partner and customer trust due to negative public perception, adverse media coverage, or client dissatisfaction. It can arise from other dangers, including fraud, data breaches, poor customer service, or unethical behavior. As a result, it has long-lasting impacts on a bank's business, client relationships, and market position.
Key Use Cases of AI in Risk Management
Artificial intelligence is crucial to risk management across various industries, including the banking sector. Here are key use cases for AI:
Fraud Detection
AI works with fraud detection, analyzing large volumes of transactional data and identifying patterns indicative of fraudulent activities. It detects anomalies, unusual behaviors, and suspicious transactions in real time. As a result, banks mitigate fraud dangers effectively.
JPMorgan's fraud detection technology has advanced over time, starting with simple rules and decision trees. But now, they employ machine learning techniques to extract important information like company and person names from unorganized data — and analyze it to identify potential fraud. Also, they utilize large language models to spot any indications of compromise in emails.
Credit Risk Assessment
How can banks make more accurate lending decisions and manage credit risk effectively? They should use AI-powered models to examine diverse data sources, including financial statements, credit histories, and market trends.
Bank of America Corp. has started utilizing artificial intelligence (AI) to forecast the likelihood of companies’ default. They are now employing natural language processing to analyze transcripts of earnings calls. By doing so, they can estimate the likelihood of companies defaulting within the next year.
Anti-Money Laundering (AML)
What about AML compliance? AI in banking identifies potential money laundering activities by analyzing transactional patterns, customer behavior, and risk indicators. It helps firms flag suspicious transactions and prevent illicit financial activities.
Google Cloud introduced Anti Money Laundering AI (AML AI) to combat financial crimes. One of their customers, HSBC, discovered that this new technology enables them to identify two to four times more genuine risks. Also, the number of alerts that need investigation decreases by over 60%, saving valuable time and reducing effort.
Cybersecurity
The development of an AI risk management helps detect and respond to cyber threats in real time. The technology uses machine learning algorithms to detect abnormal network behavior, identify malware, and safeguard sensitive data against cyber-attacks.
Qatari banks are increasingly using AI-based systems such as Tessian and Expert System, motivated by a rise in cyber-attacks carried out through emails. Additionally, some of them utilize a genetic algorithm to perform scalable analysis of their network traffic.
Market Risk Analysis
AI has proven itself well as a marketer as well. It studies market data, news feeds, social media, and other relevant information to assess market trends, conduct sentiment analysis, and predict potential dangers. As a result, banks can make more informed decisions.
Operational Risk Management
AI simplifies and improves operational risk management by finding possible weaknesses, studying past data for patterns, and offering valuable insights. Banks use AI tools to prevent operational disruptions and improve overall risk-handling techniques.
Deutsche Bank and NVIDIA are collaborating to test a group of powerful language models called Financial Transformers. These models identify early warning signs related to the other party involved in a financial transaction. They also enable faster retrieval of data and assist in spotting any issues with data quality.
Benefits of AI in Banking Risk Management
AI brings several benefits to banking risk management, including:
Improved risk assessment
AI algorithms analyze data for more accurate and timely risk assessments. This helps banks identify potential hazards, evaluate their impact, and make informed decisions to mitigate them effectively.
Enhanced fraud detection
AI in the banking market detects and prevents fraudulent activities by studying patterns, anomalies, and unusual behaviors in real time. This reduces financial losses and protects customers' funds and sensitive information.
Real-time monitoring
AI enables continuous monitoring of transactions, activities, and market data, allowing banks to identify and respond to emerging dangers promptly. This enhances risk management capabilities and helps prevent potential threats.
Compliance automation
AI technology automates compliance processes, ensuring adherence to regulatory requirements such as anti-money laundering (AML) and know-your-customer (KYC) regulations. It reduces manual efforts, improves accuracy, and mitigates compliance risks.
Efficient data analysis
Banks use AI to process and analyze large volumes of structured and unstructured data, including financial reports, market trends, customer data, and regulatory documents. This enables firms to derive valuable insights for risk management and decision-making.
Operational efficiency
Implementing the use of AI in banking requires considering the automation of manual processes, such as data entry, document analysis, and repetitive tasks. This improves operational efficiency and reduces human errors, freeing resources to focus on more strategic activities.
Cost Savings
By automating processes and reducing manual efforts, AI technology helps banks reduce operational costs associated with risk management. It improves efficiency, accuracy, and productivity, leading to cost savings in the long run.
Conclusion
The use of AI in the financial sector has revolutionized how financial institutions approach and mitigate dangers. Through advanced algorithms and data analysis, AI enables banks to proactively identify, assess, and manage risks more accurately and efficiently. This article explored popular use cases of AI in banking risk management, including credit risk assessment, fraud detection, and operational risk analysis.
Now it's time to harness the immense potential of AI and machine learning in banking with Integrio, your trusted vendor for cutting-edge solutions. With over 20 years of experience, our dedicated team of talented data scientists, equipped with advanced Math and Computer Science degrees, is ready to transform your business.
Our solutions are designed to redefine your banking risk management, from prediction and recommendation engines to cluster analysis and many more. We understand the importance of accuracy, efficiency, and innovation in the financial sector, and we strive to deliver exceptional results that propel your business forward.
Don't miss out on the benefits of AI for existing risk management practices — contact our team.
FAQ
AI is used in risk management in banking by analyzing large amounts of data to identify patterns and anomalies that indicate potential dangers, helping companies proactively detect and mitigate them. It can also automate risk assessment processes, improving efficiency and accuracy in evaluating and managing various hazards, such as credit, market, and operational.
The applications and benefits of AI in banking include improved accuracy in identifying and predicting dangers, faster detection and response to potential threats, and enhanced efficiency in assessment processes. AI enables companies to make more informed decisions, mitigate risks proactively, and ultimately enhance their operations' overall stability and security.
The associated risks of using AI include ensuring data privacy and security, as the technology relies on vast amounts of sensitive customer information. Also, the explainable AI in credit risk management is important, as regulatory requirements and ethical considerations demand transparency in decision-making processes.
Various types of AI are used in banking, including machine learning, natural language processing (NLP), and robotic process automation (RPA). ML enables banks to analyze data and make predictions, NLP allows for language understanding and chatbot interactions, while RPA automates manual and repetitive tasks for increased efficiency.
Contact us
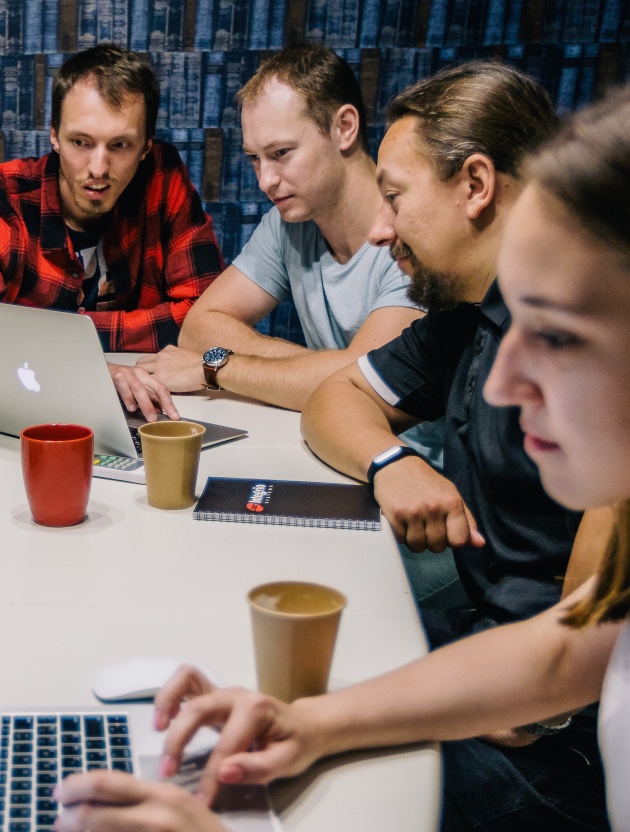