ML in Manufacturing Industry: 10 Use Cases in 2024
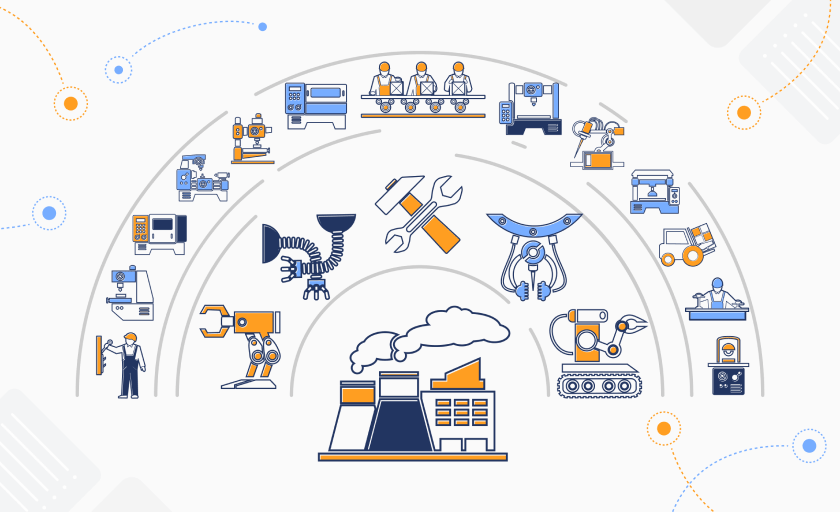
The world is entering the fourth industrial revolution, labeled "Industry 4.0". It gives rise to a new sector in manufacturing that uses machine learning technologies — Smart Manufacturing. Algorithms let businesses reduce costs, increase efficiency, and improve planning. How? In this article, we will try to figure it out by discussing the most practical ML use cases in manufacturing.
For more than 20 years, Integrio has been creating and modernizing software for manufacturing to help companies manage their processes more intelligently. Our AI/ML-driven solutions help to improve KPIs, reduce the impact of the human factor, and adhere to quality standards. In this article, we want to share our experience using ML in manufacturing.
Benefits of Machine Learning in Manufacturing
How can your business benefit from bringing ML to manufacturing? We prepared the list with the main AI/ML advantages.
Cost Reduction
One of the biggest benefits of AI/ML in manufacturing is cost reduction. It becomes possible by optimizing inventory management and supply chain, automating production tasks, preventing defects, etc. You can also plan maintenance and repairs, extending the lifecycle of your equipment.
Quality Improvement
ML algorithms recognize images and capture the slightest changes in the functioning of your equipment and systems. This allows you to find, classify, and fix defects faster. Buyers don't receive defective products, improving your reputation and reducing return and warranty costs.
Better Efficiency
ML-powered software analyzes massive datasets to gain insights and detect noteworthy patterns. Use these outcomes to optimize your processes, reduce downtime, and ensure the right level of raw materials for smooth and continuous production. As a result, you produce more with less effort.
Enhanced Worker Safety
Predictive analytics helps to learn historical data and identify patterns related to workplace injuries and incidents. Sensor systems monitor the state of equipment and the employees' health conditions. Predictive maintenance helps you identify breakdowns in time and send equipment for repair. This way, you can improve the safety of your work.
Real-Time Decision-Making
Want to make informed decisions in real time? ML will help you analyze and process data as soon as it is generated. You can quickly respond to changes, dynamically adjust production schedules, manage inventory, control quality, and reduce risk. You will also examine market data and customer behavior to maximize revenue.
Top 10 ML Use Cases In Manufacturing
There are many ways machine learning enhances your production. Let's explore the most promising AI use cases.
Generative Design
AI/ML in manufacturing lets you simplify time-consuming engineering design processes. For example, it allows you to develop new product designs considering the material, weight, strength, and cost parameters. The algorithm evaluates the layouts created and determines the most suitable ones according to your requirements. Then, if necessary, you can modify them manually.
This way, you don't only save money and time but also get access to a lot of options. The advantage of this approach is that you go beyond the usual ideas and can look at the problem from a different angle.
Collaborative Robots
Collaborative robots, or cobots, work alongside humans. They can learn different tasks, detect obstacles, and avoid them. Most often, companies use cobots for heavy lifting or on assembly lines. For example, a cobot picks up and holds a heavy part while a worker fastens it.
At the same time, special sensors make robotics safe for people, as they detect the presence and movement of a person. The intuitive interface allows any worker to program the robot as they need.
Robotic Process Automation
While companies use robots on production lines, robotic process automation (RPA) is more useful in the back office. RPA enters data, transfers information between systems, fills out forms, makes requests, and performs other repetitive tasks. This frees up employees, allowing them to focus on more strategic processes.
The technology helps companies reduce the risk of errors, increase efficiency with 24/7 availability, and boost employee motivation. RPA is effective for solving routine tasks, but it is important to correctly evaluate and select processes for automation. Some of them may require more complex software or human intervention.
Digital Twins
Want to understand the inner processes of complex equipment? Use digital twins. These virtual models of objects receive information about their physical counterparts through intelligent sensors. This way, companies can track performance by modeling and predicting problems.
For example, you can create virtual prototypes before physical production begins and identify potential problems early. Or predict the need for maintenance, preventing breakdowns and reducing downtime.
Predictive Maintenance
Today, manufacturing plants and railways are increasingly turning to predictive maintenance (PdM). Forget fixed-schedule maintenance and use algorithms to foresee a component or system failure.
It is essential to perform maintenance on time. Otherwise, this will result in severe wear or breakage, endangering workers or requiring costly repairs. By servicing too early, you waste money and other resources.
Energy Consumption
Worker robots and other technologies do not have needs like human beings. Therefore, the potential of a “factory without light” is now discussed with optimism. In addition to light, air conditioning, heating, and other familiar conditions will not be needed there. This will help companies save resources. Of course, we are talking about fully robotic factories.
The second application of ML is energy consumption forecasting. This way, you can ensure that resources are available to meet production needs. And avoid delays associated with price changes or the availability of resources in the market.
Demand Prediction
ML in manufacturing industry can detect patterns in the behavior of the target audience and provide valuable information to producers. It includes historical sales and competitors' data, seasonality, prices, promotions, market economics, etc.
With a projected increase in demand, companies can grow their production of goods. With its decrease, they will reduce the volume and switch to other goods. Thus, you optimize the development process, stocks, and supply chains. The outcome? Waste reduction, cost savings, and customer satisfaction.
Inventory Management
Rely on machine learning algorithms to better manage your inventory needs. In addition to predicting demand, the technology tracks stocks and sends out alerts in a timely manner when they need to be replenished. To make it real, it is vital to integrate the inventory management platform with enterprise resource planning (ERP) and supply chain management (SCM) systems.
ML is also useful in identifying bottlenecks in supply chains, such as supplier performance, weather, or market trends. Algorithms predict whether an ingredient will be delivered on time and how its delay will affect production.
Supply Chain Management
Manual supply chain management can drive any manufacturer crazy, as you deal with many orders, purchases, suppliers, and materials. ML is indispensable here, especially when it comes to defects.
Let's say you receive components from two suppliers, one of which sent you a defective batch. You need to track down all the products that were produced with parts from this batch so that you can act immediately. ML helps you quickly identify the required items, saving your reputation and money.
Error Detection
Accelerate the detection of manufacturing errors early, before going to market. ML algorithms uncover deviations from normal product features or functioning. Moreover, with their help, you can identify the root causes of errors by analyzing historical data and process parameters, improving production.
Using computer vision, which evaluates images and videos to identify defects, is also helpful. This way, you discover what is invisible to the human eye, especially regarding small details in technologically complex products.
The Future of ML in Manufacturing
What will the future of machine learning in manufacturing look like? First, we will observe the growing number of autonomous factories that operate with little or no human intervention. Machines and equipment will be able to connect and optimize themselves.
The models will integrate data from different sources as everything will have IoT devices, sensors, and video streaming capabilities. At the same time, insights and conclusions from AI will become more understandable for the everyday worker.
Products will become more personalized, considering customers' needs and preferences. And digital twins, which are already contributing to production, will help ensure their reliability. Manufacturing defects will be reduced to zero with predictive analytics and real-time quality control.
And, of course, the sustainability of production will increase. ML will optimize energy consumption as much as possible, reduce waste, and minimize environmental impact.
Boost Your Manufacturing with Integrio
Machine learning has forever changed the way companies do manufacturing. Now you can robotize production and the back office, design goods faster, predict demand, detect errors, and improve inventory and supply chain management. This will help you increase efficiency, reduce costs, and improve quality.
Integrio creates innovative AI/ML solutions, including prediction engines, cluster analysis, forecasting, etc. Our data scientists have PhDs in Math and Computer Science, as well as extensive experience in manufacturing. ML-powered software will help you optimize productivity, lower costs, predict risks, and profit faster.
Contact Integrio to experience all the benefits of machine learning in manufacturing
FAQ
Machine learning (ML) is used for various applications, including predictive maintenance to identify potential equipment failures, quality control to detect defects in real time, and process optimization to improve operational efficiency. Algorithms analyze large datasets, extract patterns, and make data-driven decisions, reducing downtime and optimizing production.
ML improves productivity by optimizing manufacturing processes, foreseeing equipment failures, and reducing downtime. With predictive maintenance and real-time analytics, companies can proactively address potential issues, minimize unplanned downtime, and improve overall efficiency.
Nissan and Volkswagen are using machine learning to create effective designs. General Electric's Predix Operations Performance Management solution optimizes plant productivity and throughput. And Siemens implements predictive maintenance to reduce downtime and ensure product quality.
The benefits of machine learning go beyond specific companies and affect the global industrial landscape. For example, the widespread adoption of ML in manufacturing has far-reaching implications for economic development, resource management, sustainable development, and global cooperation.
Contact us
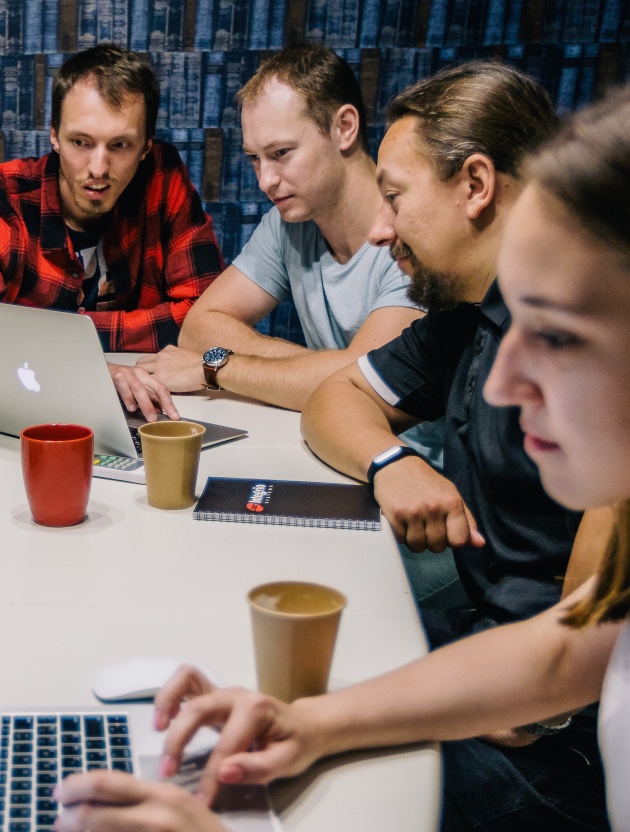