AI in Travel Platforms: Chief Use Cases in 2024
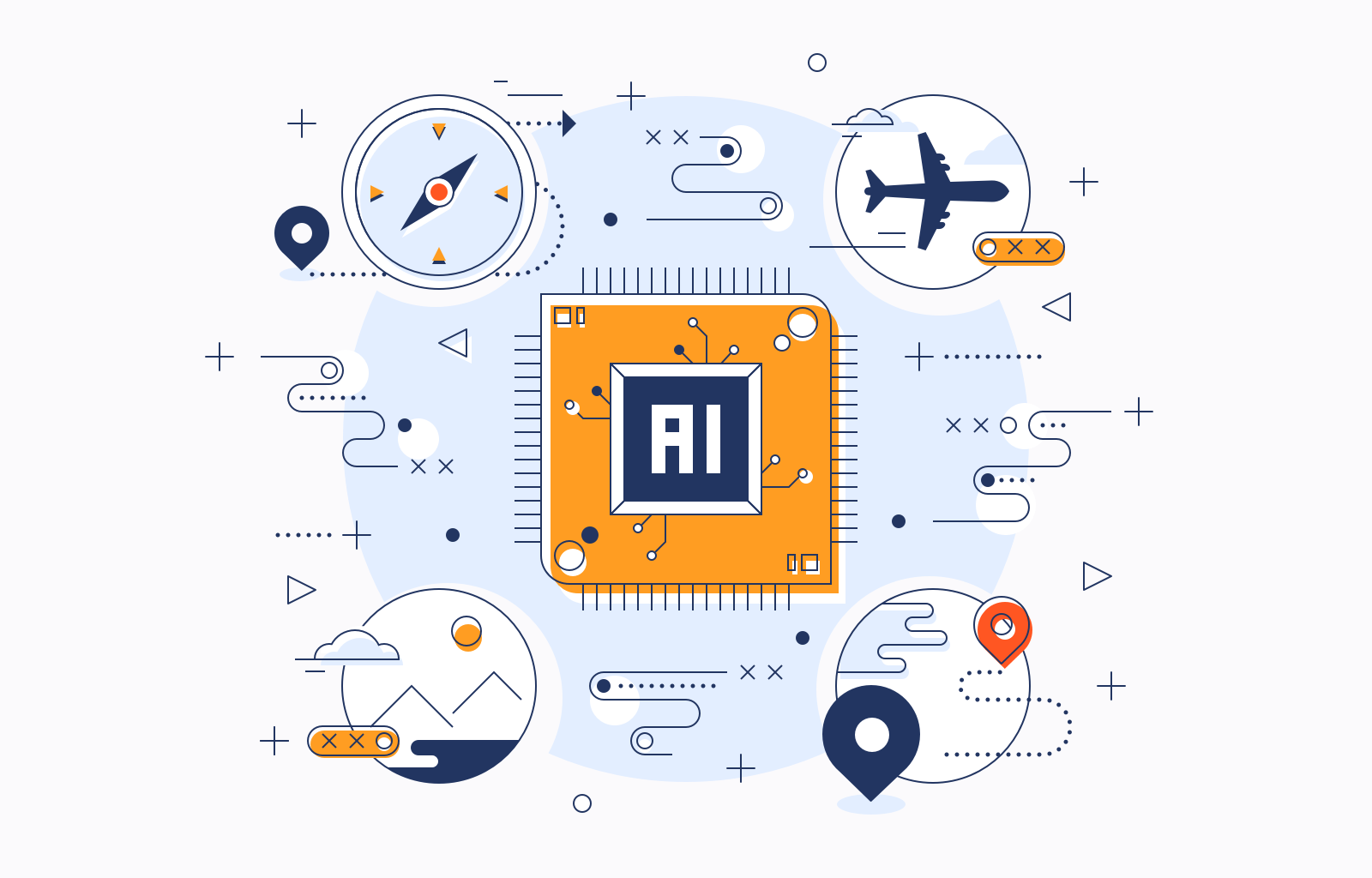
Artificial intelligence (AI) truly is everywhere. Retail, telecommunications, real estate, healthcare, and many other sectors leverage the capabilities of machine learning to enhance products and profits. In this article, we explore the foremost use cases and some real-life examples of AI put to work in travel platforms. Why look at the travel industry, specifically? In 2024, we’re all living in the world COVID created, but this is even more true for travel. The travel sector is still recovering from the global pandemic, and AI can not only assist in that recovery, but spur the industry’s overall development and betterment.
Recommendations
Data science shapes our daily lives in many ways, but one of the most familiar is through recommendation algorithms. If you’re reading this, you’re almost certainly accustomed to seeing the “Recommended for you” sections on YouTube, Amazon, Instagram, and other sites you use daily.
Recommendation algorithms are no less powerful on travel platforms. They can analyze registered users'booking and search history to offer relevant suggestions for hotels, flights, packages, destinations, activities, and specific events such as festivals or conferences.
High-quality recommendations can broaden customers’ horizons, showing them easily overlooked opportunities in the niches they prefer or even prompting them to consider new adventures that match their interests in surprising ways.
For unregistered users who don’t have an interaction history within the website, spot recommendations can still enhance their experience using only activity from the current session.
In this approach, an algorithm analyzes all users’ sessions and identifies what terms or products are most often searched for together. Since the recommendations aren’t personalized, they’ll never be as closely tailored to a given user as personalized ones would be. Nevertheless, tools like market basket analysis make it possible to extract powerful results from limited data.
Segmentation and clustering
Segmentation and clustering are two more machine learning techniques with a lot to offer travel platforms. Though less familiar to the average user than recommendations, segmentation and clustering help businesses engage their audiences better and provide better user experiences and better marketing returns.
The goal of segmentation and clustering algorithms is to separate users into groups that allow vendors to target promotions, marketing campaigns, and sales to the customers most likely to respond to them. In general, these techniques should be applied to registered user data. For example, if data analysis identifies a group of users who ski regularly or are most likely to travel in order to ski, this is the perfect audience to whom to promote ski resorts.
The more sophisticated the inputs and grouping techniques employed, the better the insight into users’ preferences. In this way, travel platforms can implement clustering or segmentation that responds to their current business needs or goals and identify the most fruitful group of customers to engage.
Natural language processing
Since the release of ChatGPT, natural language processing (NLP) has become a household term. Enterprises the world over have hastened to leverage this powerful tool.
For travel platforms, the obvious application for this branch of AI is in chatbots. Chatbots, often called virtual assistants, can provide customers with targeted recommendations by prompting them to provide parameters such as their travel dates, price range, and destination. They can also answer questions about a visa application process, provide information about travel insurance, and recommend (or provide) concierge services. Moreover, chatbots can provide information not only about upcoming trips, but about a given travel agency, raising customers’ awareness of the company’s history, competitive edge, and special offers.
Another application for NLP is in analyzing user sentiment. These algorithms can analyze social media mentions, reviews, and other feedback to understand trends in users’ wants and expectations. Armed with that crucial information, businesses can adapt their strategy and be prepared for upcoming changes. Similarly, NLP can identify positive or negative experiences from users’ feedback. Customer satisfaction is the ultimate test of any venture, and reliable insight into your company’s reputation can identify areas for improvement, highlight opportunities for expansion, and fuel growth.
Lifetime prediction and churn analysis
Two more techniques can deliver valuable insights from registered user data: lifetime prediction and churn analysis.
Churn analysis helps businesses understand customer loss rates so that they can understand, predict, and ultimately reduce that churn. Using registered users’ booking and browsing history as inputs, a certain family of machine learning algorithms can determine customer churn and lifetime, which in turn allows companies to reengage inactive or near-inactive users with effective marketing campaigns and promotions.
Dynamic pricing
Finally, machine learning lets businesses offer savvy and competitive dynamic pricing. By analyzing seasonality, demand, location, and possibly even competitors’ offers, an AI tool can suggest the optimal price for travel packages, rentals, and other activities or services. This helps companies keep their finger on the pulse of price trends while attracting new customers with options that are attractive and current.
Real use cases
The biggest names in the travel industry are incorporating AI in their enterprise life cycle already.
Tripadvisor uses an AI-powered system to make travel itinerary recommendations, while travel search platform Kayak uses machine learning for price comparison: their algorithm analyzes hotel and flight price data to find customers the best deals on their trip. HotelMize also uses machine learning to offer dynamic pricing.
Expedia, Skyscanner, Kayak, and Booking.com have implemented ChatGPT-like chatbots to engage their customers instantly and effortlessly, right from the front page. Where once these virtual assistants were a novelty, they’re fast becoming a necessity as users come to rely on and expect them.
The Dorchester Collection of hotels uses AI algorithms to analyze customers’ feedback and gauge its overall performance (and with these tools, identified deficits in loyalty to some of its hotels). Similarly, PaxPulse analyzes user sentiment and generates recommendations to resolve concerns.
Conclusion
Here, we’ve taken only a peek at some of the many applications of AI to travel platforms – especially as the travel sector and machine learning discipline develop a deeper understanding of each other and discover new opportunities for collaboration every day.
So be creative: today’s travel world has plenty of space for inspired ideas and nonstandard solutions. Maybe yours is the one with the potential to change it.
Contact us
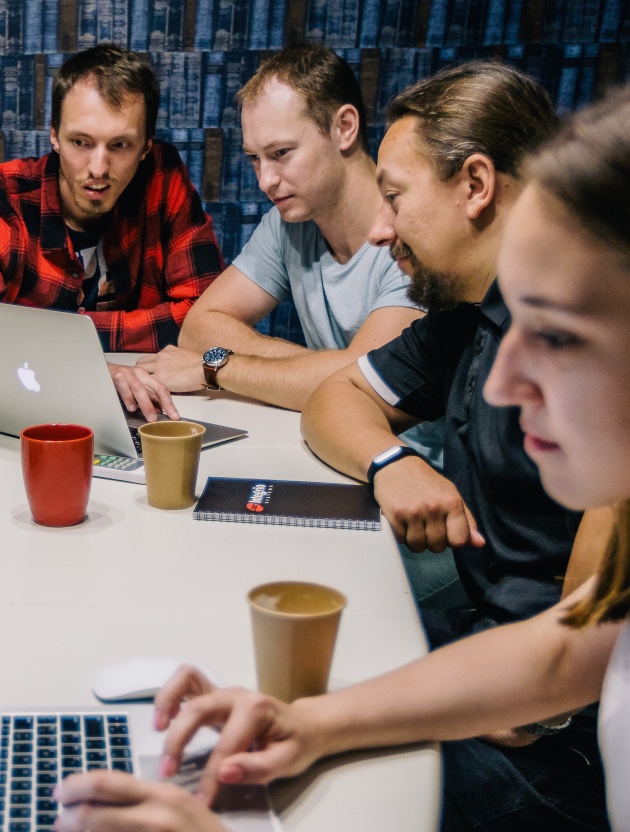