Cloud-Based Analytics
The Big Data revolution always came with one sizable problem - how to get the vast datasets running through a company's system working together quickly and efficiently? And how can all this data be used by an analytics department so that models built won't produce bad data or even go stale?
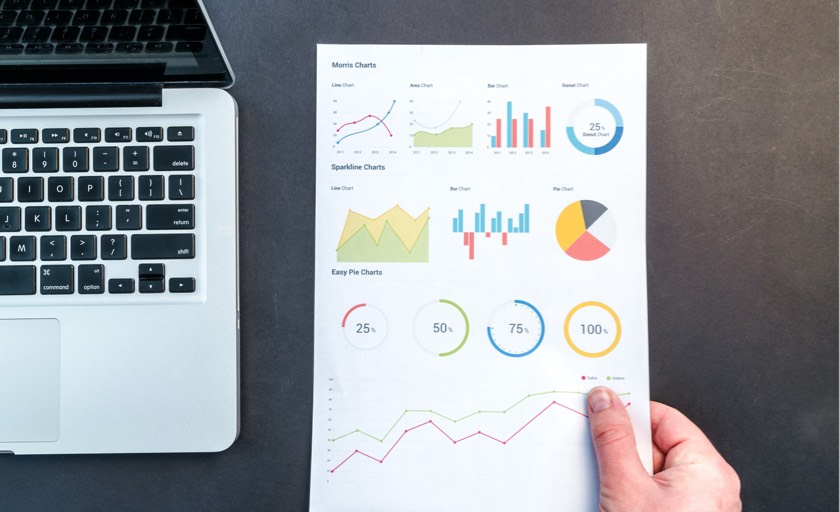
Used at the most opportune and valuable time, data can be priceless. Used an hour after that, it can be worthless. It is extremely tricky to capture data, cleanse it, transform it, model it, then send it to the people best able to utilize it and extract value from it. Even when real-time streaming data isn't involved, the cloud is often the best option; when the data is flowing through social or marketing channels, the cloud is a necessity. It makes finding, acting, modeling, and reacting to data easy and instant, something that cloudless systems struggle with.
Big Data
The term "Big Data" is everywhere these days, and it has become a kind of catch-all for data of all kinds, including analytics, the Internet of Things (IoT), social media, real-time streaming, as well as many other forms of data. Big Data utilizes this data in analytical techniques like AI, association, classification, cluster analysis, data mining, deep learning, machine learning, network analysis, optimization, regression, rule learning, text analytics, and time series analysis.
Cloud analytics has revolutionized big data and business intelligence
- Cloud analytics offers a unified approach to data, allowing fast access to data to all those who need it, which can effectively create a deep cross-organizational integration of data. A cloud analytics platform centralizes data use and data access, which makes it easier to secure and ensures its authenticity. Data stored and analyzed in a cloud increases data collaboration, increasing its value substantially.
- Cloud analytics empowers businesses to run more efficiently. It increases collaboration between departments, adds strong layers of security, scales almost infinitely as data storage and data analysis can be added as needed, and provides decision-makers with much faster access to business intelligence. Deep data granularity provides organizations with strong data governance as easily accessed audit trails show who utilized the data for what purpose, as well as what resulted from the use.
- Cloud analytics simplifies the cleansing process. Analytics are useless with an underlying data environment that is filled with poor-quality data. Any resulting models will be useless; "junk in, junk out", as most analysts warn, and it's not a warning to be taken lightly. The quality and quantity of the data gathered will have a direct effect on the accuracy of any predictive and/or prescriptive model. Deep attention must be paid to ensure any data used by a model is prepped, cleansed, and formatted properly, or nothing of value will be created, no matter how fast and/or robust a cloud analytics solution might be.
Analytics Platforms
There are a wide array of cloud analytics programs available, all with their own unique advantages and disadvantages, including:
- Microsoft's Azure Stream Analytics - a serverless scalable event processing engine that lets users process complex analytics on their data, including real-time data. Stream Analytics supports data from Azure Event Hubs, Azure IoT Hubs, and Azure Blob Storage, and real-time dashboards can surface this data utilizing Microsoft's Power BI.
- AWS Analytics for Amazon Web Services - AWS Elasticsearch can be used for operational analytics for general monitoring, event log, or clickstream analytics. AWS supports SQL, several other programming languages and uses open APIs to ensure flexibility.
- IBM Cognos Analytics - a BI and self-service analytics platform that utilizes AI and machine learning to uncover patterns of information to provide actionable intelligence through a natural language-powered AI assistant. Cognos Analytics provides the best chart-type recommendations and includes geospatial-mapping capabilities.
- Qlik - provided by the BI specialist Qlik, Qlik Sense has both hybrid cloud and multi-cloud setups, which include various configuration options and utilizes Qlik's powerful associative engine. Qlik Sense also uses AI and machine learning in its Qlik InsightBot solution, which provides answers to user's questions through natural language processing.
- Domo - a BI and data visualization specialist, users can manage all functions from the main dashboard and the data can be accessed in real-time. Domo has its own App Store filled with paid and free connectors as well.
- Looker - Owned by Google, Looker allows users to connect, analyze, and visualize data across Google Cloud, Azure, and AWS.
- Zoho Analytics - easy-to-use dashboards that give users the ability to load data into their reporting center with just a few clicks. Users can then easily share data and collaborate on tables and reports. Licensing packages are customizable according to the number of users, rows, and data connectors.
To sum up
Cloud analytics can provide huge advantages over on-premises solutions, including lowering costs, speeding up processes, providing almost unlimited scalability and storage capacity, as well as low maintenance costs.
The cloud is the perfect place to host a company's analytics platform. The decision to move to the cloud should be a consideration for most companies as so many analytics platforms are now available on the cloud, many offering plug-and-play capabilities that include a huge variety of applications and/or connectors that can ensure organizations get the most out of their Big Data.
Contact us
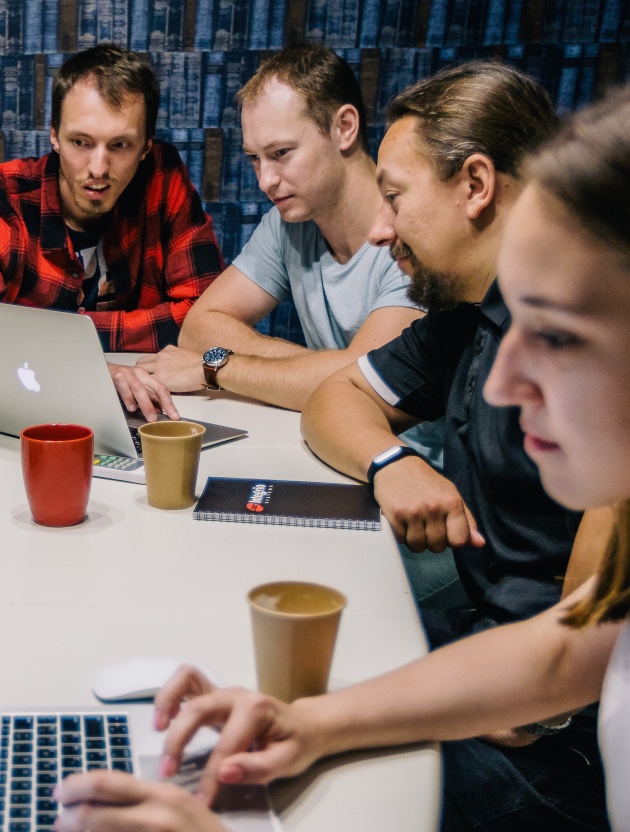